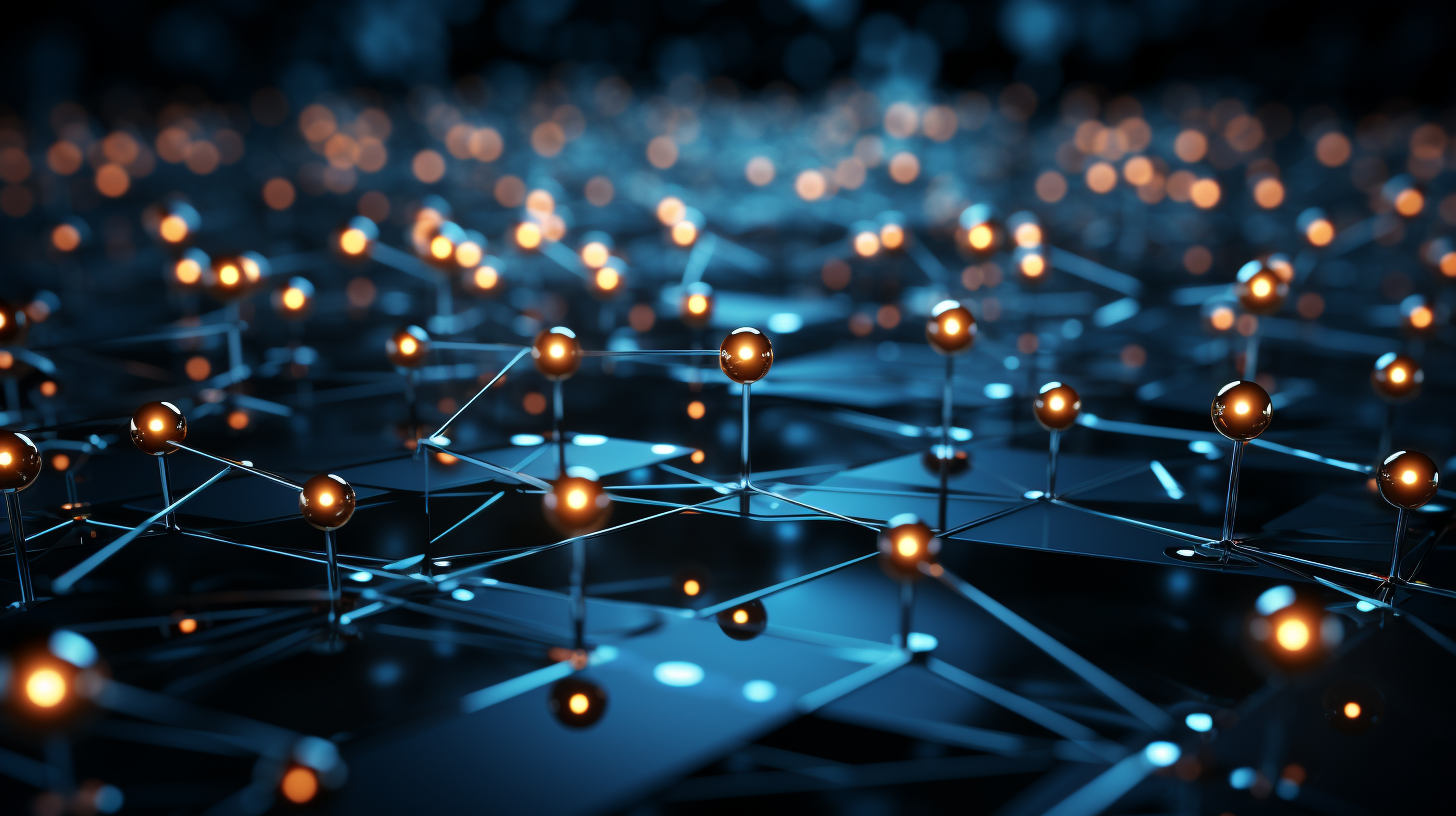
THE BLOG
The future of commercial real estate is highly predictable …..
Midjourney / Antony Slumbers
Combine the need for:
Flexibility
Adaptability
Affordability
Sustainability
Health & Well Being
Productivity
With:
Pervasive connectivity
Exponential data growth
Advanced technologies
Generative (and non) AI
And you get:
Distributed working
More flexible, project-based, and collaborative work structures
Automated administration
Higher value human to human connections
Human + Machine workflows
Ecosystems over companies
Networks over defined spaces
Hyper productivity
Looser definitions of live/work/play
Leading to:
CBDs turning to CSDs (central social districts)
More mixed use development/neighbourhoods
Growth of great and/or liveable/walkable/affordable cities
Death of dull - cities, neighbourhoods, regions
Rise of suburban, near home, third party ‘places of work’
Preference for ‘latest/greatest’ buildings - flight to quality
As strong ‘flight to character’ - aesthetics matters
Focus on ‘catalysing human skills’
Human-centricity
Culminating in:
#SpaceAsAService as the defining characteristic of the modern ‘place of work’.
Not a niche within the real estate market, but THE market.
Form follows Function follows Technology.
Agree? Disagree? It’s complicated?
#GenerativeAIforRealEstatePeople
Mega Myth - To use AI you need a lot of Data
Midjourney / Antony Slumbers
It is generally assumed that to leverage AI you need to have a lot of data at your disposal. No data = no play! This is such a prevalent belief it’s almost assumed to be a Law of Nature. I’m sure you have heard and read it a million times.
But it is not true.
At least it is not when you are dealing with Generative AI.
With Predictive AI it most certainly is, as that is all about predicting, clustering and classifying. You are applying these actions to specific datasets. So obviously without data you are facing a brick wall.
But with Generative AI the data comes built in. Take ChatGPT - the GPT stands for Generative Pre-trained Transformer, where the training has already taken place on the near entirety of data that exists on the open Internet. And from that vast corpus of data has been developed a statistical model that allows for the creation (the generative bit) of new text, code, images, video, speech or actions.
So when you are using generative AI you have at your disposal if not ‘all the worlds information’ then pretty close to it. You have vast amounts of data at your beck and call. You can, if you have it available, augment this with proprietary data, but to a large degree that is not necessary, or at least does not bring as much as you think to the party. Your data is pretty small compared to what the ‘Large Language Model’ already has intrinsically.
The bottom line is that there is a huge amount you can do with Generative AI without the need for any other data. This is what is not generally appreciated and is why this technology is also known by a different interpretation of the acronym GPT - a General Purpose Technology. Which signifies, like electricity, the internal combustion engine, the Internet itself, a technology that is not a point solution but one that is or will become pervasive throughout society. Generative AI will seep in, often invisibly, to everything. There is little you will be doing within a few years that is not, in one way or another, mediated through AI.
Indeed, there is no need to wait. Below are examples of use cases, by business department, you can implement today. No data required.
McKinsey reckon 75% of potential productivity value and gains will come from the first four categories, but the others are included to show just how much is possible ‘out of the box’. Much of this can be achieved by an individual using public tools like ChatGPT, Claude, Google Gemini and Midjourney. Whilst other areas might require customised products or coding. But either way, almost all of this is available to anyone in your company. And again, with no data required.
Sales & Marketing
Content Creation: Generate engaging marketing copy, blog posts, and social media content.
Email Campaigns: Craft personalised email messages for different customer segments.
Market Analysis: Summarise market trends and news from publicly available sources.
Customer Segmentation: Predict customer preferences using open-source demographic data.
Product Recommendations: Suggest products based on general market trends.
Interactive Content: Create dynamic web content to enhance user engagement.
Predictive Analytics: Analyse customer behaviour for better targeting and segmentation.
Product and R&D
Idea Generation: Brainstorm product ideas based on market analysis.
Prototype Testing: Simulate user feedback on prototypes with AI-generated personas.
Research Summarisation: Compile relevant research to support R&D.
Competitive Analysis: Analyse competitors' product strategies.
Design Optimisation: Propose product design improvements using generative models.
Material Research: Summarise findings on new materials from public databases.
Customer Operations
Chatbots and Virtual Assistants: Implement AI-driven chatbots for customer support.
Feedback Analysis: Analyse customer feedback from public reviews.
FAQ Generation: Automatically generate FAQ content.
Operational Efficiency: Optimise workflows to manage high-volume periods.
Personalisation: Personalise interactions based on behaviour trends.
Software Engineering (Product Development and Corporate IT)
Code Generation: Generate boilerplate code and documentation.
Bug Fixing: Identify potential bugs using publicly available datasets.
Automated Testing: Adjust tests automatically to application changes.
Architecture Design: Suggest improvements based on public best practices.
Security Vulnerability Identification: Identify vulnerabilities from public databases.
Strategy
Trend Analysis: Identify emerging industry trends.
Scenario Planning: Generate business scenarios for planning.
Benchmarking: Benchmark against industry standards.
Innovation Tracking: Track industry innovation trends.
Strategic Diversification: Analyse potential diversification areas.
Legal
Contract Generation: Generate standard legal documents.
Legal Research: Summarise legal precedents from public databases.
Compliance Monitoring: Track changes in laws and regulations.
Dispute Resolution: Suggest resolutions based on similar public cases.
Policy Development: Analyse public compliance standards for internal policies.
Risk and Compliance
Regulatory Compliance Tracking: Monitor regulatory changes.
Risk Assessment: Conduct assessments based on public threat data.
Fraud Detection: Detect fraudulent activity patterns.
Ethical Compliance Monitoring: Monitor public sentiment for ethical issues.
Cyber Risk Analysis: Analyse public data on cyber threats.
Talent and HR
Resume Screening: Automate initial resume screening.
Employee Engagement: Analyse engagement trends to inform strategies.
Training Programs: Develop AI-driven training programs.
Workforce Planning: Use labour market trends for planning.
Diversity and Inclusion: Inform policies based on diversity data analysis.
So, as you can see, it’s time to bury the ‘you need data’ myth. You absolutely do to get the most out of a lot of AI, but with Generative AI the biggest constraint is not data, but your own curiosity, vision and willingness to just get stuck in.
Using AI to Turbo Charge Human-Centric Real Estate
Antony Slumbers & Midjourney
We are all aware of just how much Covid has transformed our collective attitudes towards work and the office. Four years after the start of Covid managers around the world are still struggling with how to assimilate into their operating procedures the desire amongst the vast majority of employees to not give up what they learnt was possible during the pandemic. That being in the office five days a week was absolutely not necessary, and that the costs and constraints around commuting into a workplace are self imposed and injurious to a better way of working and living.
This is still playing out, but the end point has been inevitable for years. Post Covid was never going to be a resumption of life pre Covid. We’ve all learnt too much.
We are now in the era of human-centric real estate. Unless the spaces and places we are being asked to spend our time are conducive to enabling people to be happy, healthy and productive they will increasingly become obsolete. They will no longer have any sort of product/market fit. Indeed we are already seeing this in the market - pitiful occupancy and utilisation levels are directly correlated to having lost any sort of human utility.
All of this is a known known. What is far less understood is just how much AI is going to:
Enable the creation of human-centric spaces and places.
Make these types of spaces even more important, as the ‘work we do’ is fundamentally reconfigured due to technological change.
Provide the tools for either existing companies, or new entrants, to be dramatically more productive than is the norm today.
In the following videos I dive deep into these rabbit holes and provide frameworks for how to utilise AI to create great human-centric real estate, how to rethink your own products and services and their delivery, and how to create a company culture appropriate to this new world.
In Part 1 of this video series I am going to introduce the subject, Define Human-Centric Real Estate, and explain why we need to care.
In Part 2 of this video series I am going explain what AI can actually do to impact the six pillars of human centric real estate, and what are the the direct and indirect financial benefits of this approach?
In Part 3 of this video series I am going to look at how we’d approach this topic if we were starting from scratch, and how productive that could make us.
And finally in Part 4 of this video series I am going to analyse the culture of a human-centric company, and wrap up everything in a set of conclusions.
Shaping the AI Economy: A Holistic Approach Beyond Zero-Sum Thinking
There is much commentary that AI is likely to enable fewer people to generate a given amount of economic output and therefore large numbers of people are going to be redundant, leading to a bifurcated, deeply unequal world, where a few do exceptionally well whilst the rest see their skills commoditised and downgraded in value.
Is this right?
I think not, because it overlooks the inherently dynamic nature of economies, particularly in response to transformative technologies. It harks back to the late 19th century lump of labor fallacy which considered there only to be a finite amount of work within an economy that can be distributed to create more or fewer jobs. That proved wrong then, and I hope and suspect it will prove wrong in the future.
How does the dynamic nature of economies play out?
In six ways:
Productivity Gains and Income Redistribution:
Increased Corporate Profits: AI-driven productivity improvements can lead to increased profits for businesses. Ideally, a portion of these profits can be redistributed to workers in the form of higher wages or reinvested into the business to stimulate further growth.
Consumer Spending: Higher incomes for workers can lead to increased consumer spending, which in turn stimulates demand across various sectors of the economy, creating a multiplier effect.
2. Cost Reduction and Price Elasticity:
Lower Production Costs: AI can significantly reduce production costs, leading to lower prices for goods and services.
Increased Demand: Lower prices often lead to increased demand (price elasticity). This increased demand can stimulate the need for more diverse services and products, leading to new job opportunities.
3. Investment in Innovation and R&D:
Reinvestment of Profits: The savings and profits garnered from AI integration can be reinvested into research and development, sparking innovation and the development of new products and industries.
Job Creation in New Sectors: This innovation can lead to the creation of entirely new sectors, which require human capital, thus generating new job opportunities.
4. Shift Toward High-Value Jobs:
Upgrading Skill Sets: As AI takes over routine tasks, there is a shift in the job market towards more complex and high-value tasks that require human input, such as strategic planning, creative problem-solving, and emotional intelligence.
Higher Value-Add per Employee: This shift can increase the value added per employee, leading to overall economic growth.
5. Global Economic Integration:
Opening New Markets: AI can break down barriers to entering global markets, allowing businesses to expand their reach and tap into new customer bases.
Global Supply Chains and Trade: Enhanced global integration can lead to more efficient supply chains and increased trade, boosting global economic activity.
6. Secondary Markets and Induced Industries:
Support and Maintenance of AI Systems: The growth in AI technology creates secondary markets, including the need for maintenance, updates, and support for these systems.
Training and Education: As AI evolves, so does the need for ongoing training and education, which itself becomes a significant sector.
So the causation of higher productivity due to AI leading inexorably to fewer jobs is not real. That does not mean it is not possible. The above IS very likely to occur but it does need to do so alongside a wide range of education and regulatory measures.
For example education systems must foster lifelong learning, teaching not just technical skills but also how to adapt, learn, and grow continuously. Adaptability is the key to thriving in an AI-driven economy, so we need to emphasise soft skills, such as critical thinking, creativity, and emotional intelligence, which become as crucial as imparting technical know-how.
As AI transforms industries, the need for re-skilling and up-skilling becomes paramount. We’ll need tailored educational programs that can help workers transition from declining sectors to emerging fields. And partnerships between educational institutions, governments, and businesses to identify skill gaps and develop targeted training programs.
And in terms of regulatory measures we’ll need comprehensive policies to support workers displaced by AI. These must include not just unemployment benefits but also access to retraining programs and job placement services. And these measures have to be proactive, anticipating changes in the labor market rather than reacting to them.
Proactive fiscal and regulatory policies can help in redistributing the gains from AI, ensuring that the benefits are widely shared across the economy. Government investment in public goods, infrastructure, and education can further stimulate economic growth.
Fostering innovation and sector development can be catalysed by governments that play a proactive role in nurturing new technology sectors through incentives, research funding, and infrastructure support. The approach has to encourage economic diversification, without which creating new job opportunities and industries will be difficult.
Entrepreneurship and Small Business Support is an imperative. We have to encourage entrepreneurship, especially in AI-enabled sectors that can drive job creation. Support can come in the form of tax incentives, grants, and access to resources. Small businesses are often more agile than larger ones and more likely to be incubators for innovative uses of AI.
Globally we need to work hard at generating collaboration and setting universal standards. AI’s impact transcends borders so international collaboration on standards, ethical guidelines, and best practices are required to create a more cohesive and responsible global approach to AI integration.
And finally regarding the role of governments we need to vastly increase public awareness and involvement in reshaping economies. Educating the public about AI’s potential and challenges is vital to ensure (hopefully) a well-informed citizenry. Public involvement in discussions around AI ‘should’ help in democratising its development and application.
And then of course we also need to think about the actions and behaviour of company management. The labour economist David Autor has written that "AI Could Help Rebuild Middle-Class Jobs” but “the key question to ask is for whom is AI a substitute and for whom is it a complement” and “We Have a Real Design Choice About How We Deploy AI”.
How companies behave, and how we as societies let them behave, is a critical factor in determining all of our futures.
All of them above has to be taken seriously. Our future is not pre-wired. We do have a lot of agency, and how AI integrates or dominates our lives is still largely up to us.
All of which is very tricky for the real estate industry. Just how many jobs are there going to be, what will people be doing, and where and what real estate are they going to need? Every answer depends on how much of the above occurs. And how.
If you see our futures determined by an AI mediated world that will inevitably lead to a society where, to quote Thucydides ‘The strong do what they can and the weak suffer what they must.’ then the amount of change will be immense, and any project you start today will very much have to pander to the ‘Strong’. The ‘weak’ will have to take what they are given.
Which feels very dystopian to me, even as I am aware many people, especially the more libertarian Silicon Valley types, see this absolutely as where we are going.
I see two other options. First, we do follow the guidance above and build a much bigger pie, with a piece for everyone. Or we either don’t try this, or it turns out that AI is going to take most of the pie regardless of its size, in which case we need to be thinking of a much more distributive, egalitarian, society where the few do not get to keep their massive gains, but they are spread far and wide.
Either way, the only guarantee is that big things are afoot, the next decade is going to be transformational, and that we really must hope for large doses of wise leadership across the globe.
And that last sentence worries the hell out of me!
Let the New Cycle Begin
There is, I think, a rather discombobulating paradox about data, in real estate.
On the one hand, so much of what is possible with modern technologies is impossible without well ordered, if not necessarily structured, data.
You simply cannot play the game without it. Without data you are effectively disenfranchised, with little to offer, and no strings to pull.
However, once you are fully enfranchised, with your data ducks all neatly arranged in a row, you need to rise above and beyond data in order to do anything really meaningful.
What really matters is qualitative. Quantitative data only gets you to base camp. It’s the qualitative that opens up the summit.
And yes, there is qualitative data, but it’s of a different character to quantitative, and mostly treated as ancillary by ‘excel jockeys’. Soft and fluffy versus hard and real.
For decades real estate has been about the quantitative, the inputs to financial models to divvy up the rankings of financial assets. Just a numbers game. Recently I heard it said that the most technologically advanced actors in real estate are those furthest away from the actual, physical real estate. When one can abstract out the physical to purely financial, data and technology is all you need.
And that has been where the most money resides. When real estate is but a financial instrument it’ll follow that the real estate is not what matters.
But when real estate becomes more about ‘social infrastructure’, as memorably termed by Gareth Lewis and the team behind this years PwC ‘Emerging Trends in Real Estate’ report, the qualitative moves to the fore. What matters when its effectiveness as a financial asset is dependant on messy, complicated, nuanced and eternally in flux human-centric variables?
Data does matter. Critically so. But it is very much necessary but not sufficient. Those financial returns will not emerge by power driving excel like in ‘the good old days’.
Layer upon layer of design, imagination, empathy, critical thinking, vision, humanity and countless other qualitatively nurturing variables are now required in the mix.
The office market is exhibit number 1. The most beaten into submission by excel real estate asset class. Sheet upon sheet of macros determining precision investing and analytical rigour. Home to the very best ‘numbers guys’ (mostly).
Which all looked so professional, authoritative and grown up. Returns guaranteed, the future mapped.
Right up until Covid demonstrated to the individual occupiers of these spaces (hitherto absolutely NOT the customer) that they really did not NEED them anymore. At least in anything like the quantity or duration that they did before.
And so they sit, under occupied and under utilised. Steaming elephants in the room. Slayers of spreadsheets. Every professional, authoritative and grown up analysis eviscerated.
Or at least that is the reality, despite being generally skirted around. 3 years of pretence that the world has not changed goes on.
And will do.
Until the penny drops. That real estate as financial instrument is a thing of the past.
When your customers are users, not financiers, and when for financiers to get what they want, the customer has to first be satisfied, the entire structure, character, dynamic and incentives of real estate needs to change. The existing ‘immune system’ has to be overwhelmed and a new order installed.
The leopard has to change its spots.
Easy? No.
Inevitable? Absolutely!
Let the new cycle begin.